The Role of Technology in Fraud Prevention
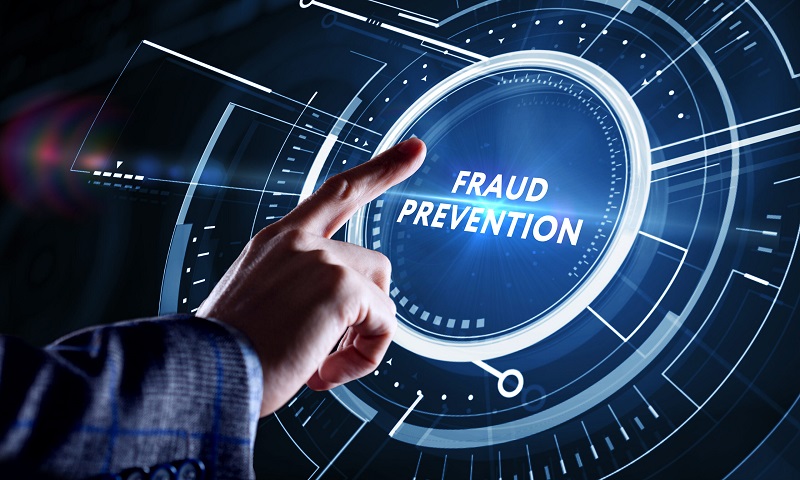
Fraud is a huge problem for all kinds of businesses. It can lead to significant financial losses. Emerging technologies like AI, data analysis and continuous monitoring, blockchain and RPA can help companies detect fraud and reduce corruption.
Strategic teams at banks are converging three domains-identity management, fraud analytics and financial crimes, to create economies of scale, improve customer experience and reduce the risk of false positives.
Big Data
Organizations can access vast unstructured data in real-time as the world becomes increasingly digital. This data can be used to identify fraud patterns and prevent security breaches before they happen. For instance, if a bank notices that a credit card transaction occurs from different locations and devices on the same day, this will raise red flags. Banks can use this information to detect and stop fraudulent activity before it occurs.
However, leveraging big data for fraud prevention requires a new approach to analyzing information.
Instead, they must employ predictive analytics systems to analyze massive volumes of structured and unstructured data in real time. These systems can also help them identify relationships between the various types of information and pinpoint anomalies.
This can help them identify and mitigate fraud, bribery and corruption, money laundering and other white collar crime before it happens. As a result, they can minimize their risk and protect their customers’ sensitive data. This will help them meet compliance obligations and avoid costly fines and penalties. The good news is that the tools needed to tackle Big Data are becoming more accessible thanks to advances in programming languages and server technology.
Artificial Intelligence
Artificial Intelligence (AI) uses data to recognize patterns and identify anomalies that could be fraudulent. It’s an automated process that works around the clock and continuously learns to find new ways. This is critical since fraudsters are constantly adapting their techniques.
AI is used in many industries to improve customer service, increase productivity, and reduce risk. For example, a business can use machine learning to detect and prevent fraud by analyzing transaction data, such as identifying red flags like multiple payments from the same account or detecting suspicious behavior in an application form. It can also help a company automate manual processes and save time. AI can translate documents into other languages and analyze data, allowing businesses to make faster decisions.
Companies can use AI to prevent financial crimes, including identity theft, phishing, unauthorized account access, money laundering, insurance fraud, etc. The technology can detect unusual patterns in transaction data and alert a user about them in real time. Fraud prevention systems can also use machine learning to predict future trends and stop them before they occur.
While numerous success stories prove the value of AI, incorporating the technology into a company requires careful planning and execution. AI projects are computationally expensive and require expertise in high demand and short supply. This is why it’s important to understand when and how to incorporate AI into a business and when to turn to a third party for help.
Machine Learning
A machine learning approach to fraud detection leverages the tremendous volumes of data, computation power affordability and high-speed Internet availability to rapidly develop and test complex models that can detect suspicious patterns. This technology provides an edge for organizations fighting a constantly evolving fraudster who has learned to work around heightened security measures.
Supervised machine learning software analyzes past fraudulent activities and determines what characteristics indicate fraud to predict future suspicious activity better. Financial institutions typically use these methods to scan documents and identify abnormalities, such as font or document format alterations, that could indicate fraud. They also help identify new types of fraud, such as phishing attacks or identity theft.
Unsupervised machine learning assesses and examines data that does not contain identified fraud using techniques such as clustering, association mining and dimension reduction. This type of analysis identifies patterns and hidden connections that reveal networks of interest. It also uses text analytics to accurately identify expressions of names, times, companies and monetary values through search, content categorization and entity extraction.
Fraudulent activity not only costs organizations billions of dollars, but it can also damage customer trust and hurt brand reputation. A single fraud case can result in fines for private businesses, regulatory violations for public entities and lost funding for nonprofits.
Data Visualization
Often, a fraud case requires connecting the hidden dots behind raw numbers. This is where data visualization comes into play. It provides intuitive ways to display connections in your data and zero in on bad actors using geospatial and heat map indicators.
It is important to keep your audience in mind when designing data visualizations. You want your insights to be easily accessible to various audiences, regardless of their level of expertise with the subject matter or BI tools. This accessibility helps you communicate your information meaningfully, which can ultimately help you drive more informed decision-making throughout your organization.
A good visualization can tell a story. It should make it easier to recognize trends and outliers that may not be immediately apparent when looking at your data alone. This helps you to identify “a-ha” moments that could lead to better operations, planning, strategies, and actions.
Fraud investigators must be able to rapidly and accurately interpret complex sequences of events and connections, especially during a pandemic when time is money. Software that automates visualization can cut months of data preparation time to a few days, speeding up an investigation and allowing fraudsters to be caught faster. By enabling investigators to quickly and accurately identify patterns, these tools can also improve the accuracy of predictive models for detecting fraudulent behavior.